Predicting fine-scale forage distribution to inform ungulate nutrition
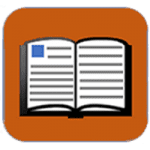
This study modelled 20 forage species that are suitable for mule deer and Rocky Mountain elk. Climatic, topographic, soil, vegetation, and disturbance variables were attributed to approximately 44.3 million habitat patches generated using multi-scale object-oriented image analysis. Lasso logistic regression was implemented to produce predictive SDMs. The study evaluated if the inclusion of distal environmental variables (i.e., indirect effects) improved model performance beyond the inclusion of proximal variables (i.e., direct physiological effect) only. Results showed that all models provided higher predictive accuracy than chance, with an average AUC across the 20 forage species of 0.84 for distal and proximal variables and 0.81 for proximal variables only. This indicated that the addition of distal variables improved model performance. The study validated the models using two independent datasets from two regions of Idaho and found that predicted forage species occurrence was on average within 10% of observed occurrence at both sites.